When statistical inference and automatic diagnosis are performed using MRI data acquired at multiple institutions, performance degradation may occur due to differences between different MRI devices and imaging protocols (measurement bias) or biological differences in the MRI measurement group at each institution (sample bias). Thus, there is a need for a "harmonization" method to eliminate such bias. Harmonization in the BMB includes harmonization of data acquisition protocols (HARP), harmonization of image preparation, homogenization of subject groups (traveling subjects), and statistical harmonization.
In conventional statistical harmonization, a statistical model that considers factors that make a difference in data (measurement bias and sample bias) as covariates - such as a general linear model (GLM), a Bayesian approach (Fortin et al., 2018; Fortin et al., 2017), and a meta-analytic approach (Okada et al., 2016; van Erp et al., 2016) - has been proposed. However, it was not possible to distinguish between the two factors (Yamashita et al., 2019). If large sample datasets are available, biasing can be eliminated using cross-facility validation with machine learning and deep learning (Nunes et al., 2018). However, this is a method for extracting clear and characteristic portions in each image data. Therefore, it is not known whether the learning result by this method can be converted (generalized) to other research data.
Travelling Subject (TS) method in the BMB is thought to be useful as a powerful technique for harmonization as a method to equalize the differences among facilities for imaging data of each facility (Fig.) (Yamashita et al., 2019). In the TS technique, subjects go to all institutions for MRI. Because the same subject data can be obtained at each institution, the sample bias of the collected data can be minimized and the measurement bias can be estimated.
In the AMED Brain ProBMI Task (https://bicr.atr.jp/decnefpro/), resting fMRI and structural MRI have been performed in multiple psychiatric patients and healthy volunteers at multiple institutions. In these institutions, nine subjects underwent repeated MRI measurements using the TS method. Measurement bias and sample bias for each participant group (Schizophrenia, depression, autism, and healthy people) can be separated from individual factors and disease-specific factors (which are the targets of disease discrimination). We found that the combination of statistical harmonization reduced measurement bias by 29% and improved the signal-to-noise ratio by 40%. The BMB will further develop and expand these prior technologies to develop harmonization technologies with higher generalization performance and accuracy.
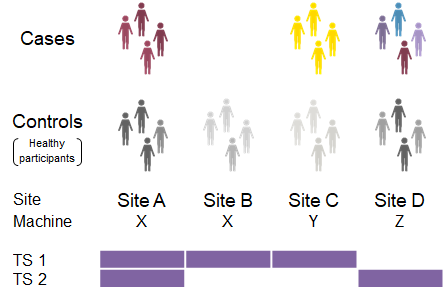
● References
-
Fortin, J. P., Cullen, N., Sheline, Y. I., Taylor, W. D., Aselcioglu, I., Cook, P. A., . . . Shinohara, R. T. (2018). Harmonization of cortical thickness measurements across scanners and sites. Neuroimage, 167, 104-120. doi:10.1016/j.neuroimage.2017.11.024
-
Fortin, J. P., Parker, D., Tunc, B., Watanabe, T., Elliott, M. A., Ruparel, K., . . . Shinohara, R. T. (2017). Harmonization of multi-site diffusion tensor imaging data. Neuroimage, 161, 149-170. doi:10.1016/j.neuroimage.2017.08.047
-
Nunes, A., Schnack, H. G., Ching, C. R. K., Agartz, I., Akudjedu, T. N., Alda, M., . . . Group, E. B. D. W. (2018). Using structural MRI to identify bipolar disorders – 13 site machine learning study in 3020 individuals from the ENIGMA Bipolar Disorders Working Group. Mol Psychiatry. doi:10.1038/s41380-018-0228-9
-
Okada, N., Fukunaga, M., Yamashita, F., Koshiyama, D., Yamamori, H., Ohi, K., . . . Hashimoto, R. (2016). Abnormal asymmetries in subcortical brain volume in schizophrenia. Mol Psychiatry, 21(10), 1460-1466. doi:10.1038/mp.2015.209
-
van Erp, T. G., Hibar, D. P., Rasmussen, J. M., Glahn, D. C., Pearlson, G. D., Andreassen, O. A., . . . Turner, J. A. (2016). Subcortical brain volume abnormalities in 2028 individuals with schizophrenia and 2540 healthy controls via the ENIGMA consortium. Mol Psychiatry, 21(4), 547-553. doi:10.1038/mp.2015.63
-
Yamashita, A., Yahata, N., Itahashi, T., Lisi, G., Yamada, T., Ichikawa, N., . . . Imamizu, H. (2019). Harmonization of resting-state functional MRI data across multiple imaging sites via the separation of site differences into sampling bias and measurement bias. PLoS Biol, 17(4), e3000042. doi:10.1371/journal.pbio.3000042